 |
|
 |
Skeleton-based segmentation and description of articulate object cyclic movements from video sequences |
Masters |
Sébastien Quirion |
Robert Bergevin (Supervisor) |
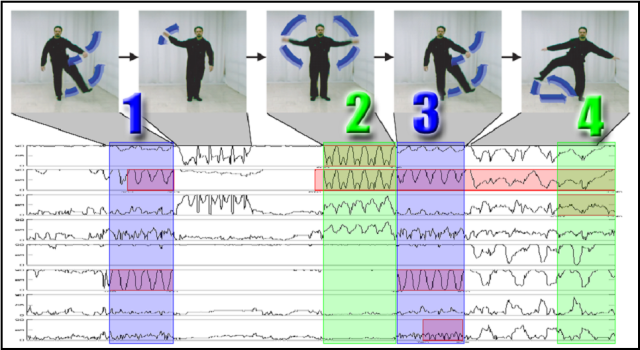 |
Problem: The recognition of activities by a computer vision system has been the subject of several research projects over the past few years and several interesting solutions have been proposed. However, the majority of this research has led to solutions which are based on the hypothesis that there is only one activity in the analyzed video sequence. In a real application, be it for a surveillance system located in an airport or an assistance system for the elderly, the analyzed video sequences can include a large number of activities which are carried out one after the other (ex. walking, running, then waving hello). We thus propose that the activities be extracted automatically from a video sequence using the information provided by a skeleton model representing the temporal evolution of a human being or other articulated object. Our method can be applied to any form of skeleton described in terms of joints linked by straight-line segments. We can thus provide sequences comprising but a single activity to the activity recognition algorithms, thereby fulfilling their first hypothesis. |
|
Approach: The proposed approach is based on a periodicity analysis of 1D signals. This allows us to use the theories of signal treatment, an area which is much better understood than that of temporal segmentation of video sequences. Our approach can be divided into four steps. First, among all signals which can be acquired from a skeleton sequence (ex. angle between two joints of the skeleton at each time, speed from the centroide of all joints, X position of a joint at each time, etc.), those 1D signals which carry more information concerning the activities carried out by the skeleton must be identified. Secondly, an algorithm enabling a robust segmentation of a 1D signal into cyclic parts must be developed. Thereafter, an algorithm must be developed which allows the segmentations obtained to be combined on all retained signals so as to obtain a unique segmentation for the treated video sequence. The final step involves providing a description of the activities isolated by the segmentation so as to indicate which signals contribute to each activity and in what proportion they contribute to these activities. |
Challenges: Even though this may seem very simple for a human being, the automatic segmentation of a video sequence into activities involves several difficulties. In order to be able to efficiently segment videos showing people, the proposed system must be robust to the small variations which a person can introduce into the different cycles of a certain activity. The system must also take into account the possibility for a person, or other articulated object, to superpose several activities (such as walking while waving). Moreover, in order not to limit the application possibilities, the system must take into account the noise of the entrance signals. This noise can be visually translated as the poor position of a skeleton with respect to the position of the subject in the original image. Depending on the adjustment process of the skeleton, this noise can have several origins. For example, the noise can come from the background subtraction process which provides a deformed silhouette or from an adjustment process of a skeleton onto a silhouette, which made lead to a poor interpretation of a silhouette and thereby adjusting an erroneous skeleton. The system must have a strong robustness towards this almost inevitable noise in the video sequences. Finally, another main challenge involves ensuring the validation of the developed algorithms. |
Applications: This research project will provide robust descriptors to classification systems and activity recognition systems. The original contribution here involves the extraction of characteristics for static recognition of shapes applied to movement analysis. |
|
Calendar: January 2004 - August 2005 |
|
|
Last modification: 2007/09/28 by squirion |
|
 |